TRAIN team members are actively advancing railway operational efficiency, performance reliability, and safety through research on various topics for a variety of railway industry and agency sponsors. TRAIN researchers develop and make extensive use of railway mainline, yard and terminal simulation models, plus detailed train performance calculators and powertrain models for various alternative energy locomotives. Selected current and recent projects are listed below.
–
Railway System Operational Performance, Capacity and Efficiency
Funding Sources: UT Austin
Maintaining a high level of service under anticipated future freight and passenger rail traffic volumes will require changes in operating strategies, infrastructure investment, and adoption of new technologies such as advanced control systems with moving blocks or autonomous trains. Making these changes and investments in an optimal manner requires a thorough understanding of the fundamental relationships between track infrastructure, traffic levels, train characteristics, level of service, operating efficiency and technology-assisted train operations. Through research, TRAIN seeks to increase knowledge of these fundamental rail system relationships within the context of both railway mainlines, and yards and terminals, and how mainlines, yards and terminals interact to determine the overall performance, capacity and efficiency of the rail network. The current focus is on using AnyLogic to simulate multiple classification yards and examine how disruptions in one yard can spread congestion to adjacent yards and the mainlines connecting these terminal facilities.
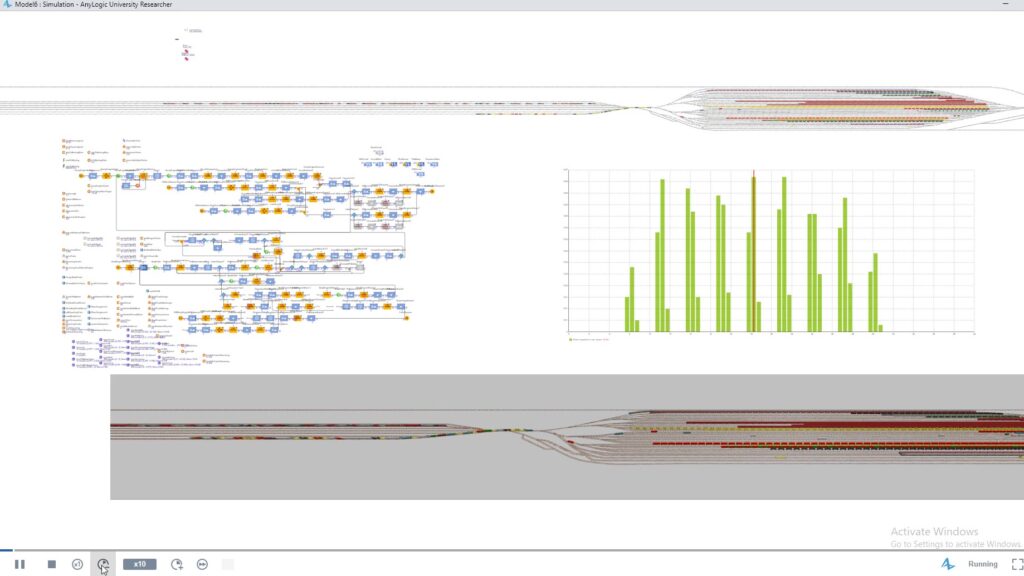
_
Cost and Benefit Risk Framework for Modern Railway Electrification Options
Funding Sources: Federal Railroad Administration (FRA)
As railroads contemplate electrification as means to reduce carbon emissions, it is worthwhile to examine the economics and technological barriers identified in past electrification proposals, and how they might be addressed by modern electrification technologies and implementation strategies. TRAIN will show how project feasibility changes with new technologies and create a risk-based framework to analyze the electrification of a given rail corridor under uncertainty in key cost and traffic parameters.
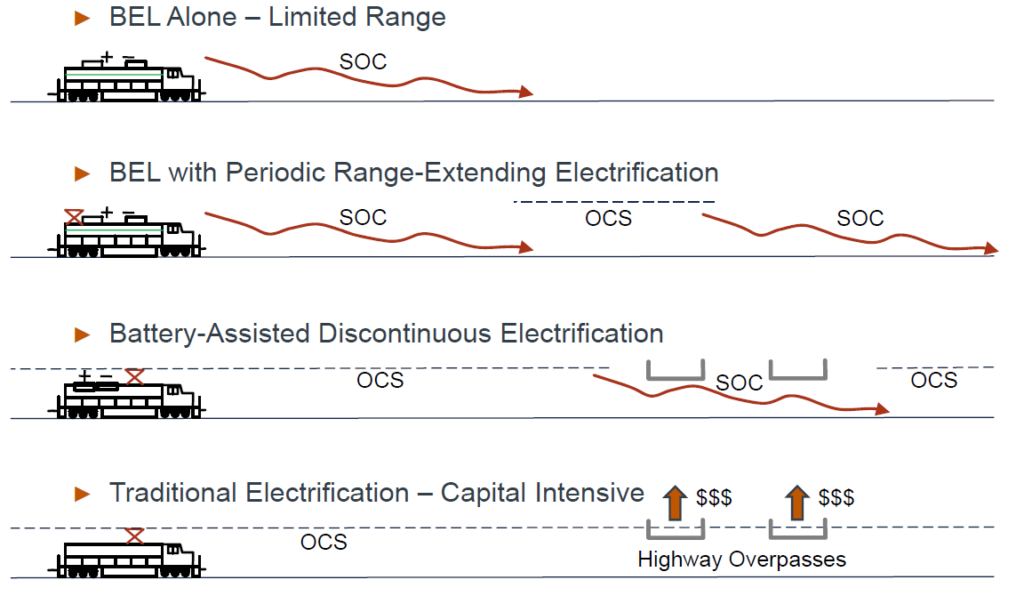
_
INFORMES: INtermodal Freight Optimization for a Resilient Mobility Energy System
Funding Sources: U.S. Department of Energy Advanced Research Projects Agency – Energy (ARPA-E); project led by the National Renewable Energy Laboratory (NREL)
INFORMES proposes to develop a national-level intermodal freight modeling framework to support decision-making in the execution and rollout strategy of decarbonization technologies and guide efficient operations of the intermodal freight system. We are developing an Infrastructure Model (IM) and a Logistics Model (LM), and integrate them in a Python-based, easily executable and publicly distributable software tool. The IM is designed to model deployment of low-carbon energy sources and systems, transshipment terminals, and emerging vehicle operations, and find the optimal spatial and temporal allocation of resources. The LM generates a robust and holistic optimization of intermodal logistics operations simulating intermodal demand on top of the stochastic transportation system.
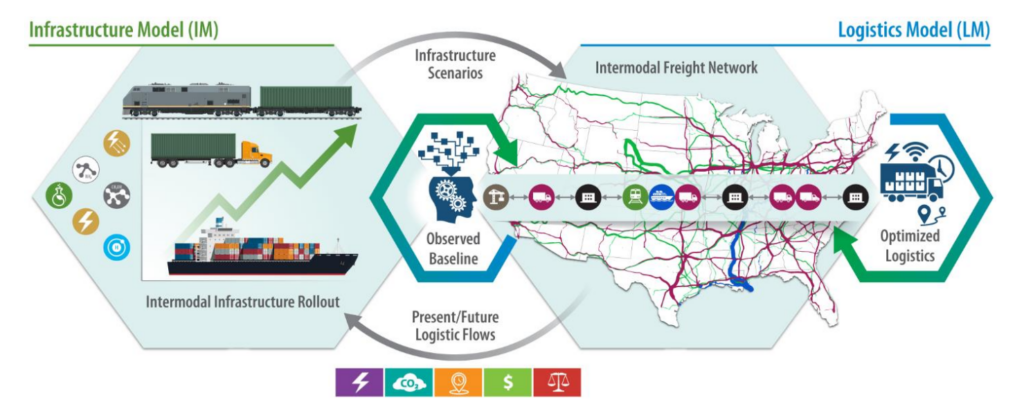
_
Transformative Rail Architecture to Decarbonize Freight
Funding Sources: U.S. Department of Energy Advanced Research Projects Agency – Energy (ARPA-E); project led by Parallel Systems and the National Renewable Energy Laboratory (NREL)
Parallel Systems (PS) is currently developing a concept to use battery-powered Self-Propelled Autonomous Railcars (SPARCs) to transport intermodal containers between ports and inland terminals with reduced emissions and improved transit times. This project will modify the previously developed Advanced Locomotive Technology and Rail Infrastructure Optimization System (ALTRIOS) to capture the characteristics of this new technology. The initial tasks require TRAIN to develop a new rolling and aerodynamic resistance model for platoons of PS vehicles. Next, TRAIN will develop a new module or sub-model to account for the PS terminal operations and allow greater flexibility in dispatching platoons into the mainline corridor simulation under traffic peaks and other sources of traffic variation. Finally, TRAIN will simulate PS platoon operations on several case study corridors to compare the performance, energy and emissions of PS vehicles to conventional intermodal trains.
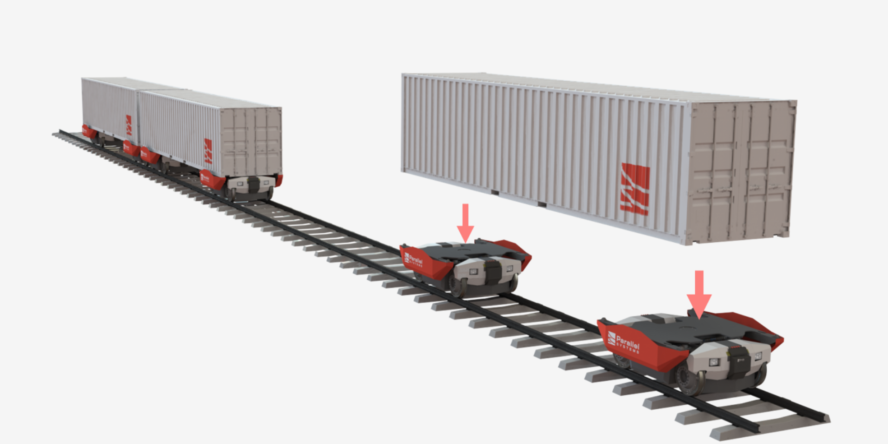
_
Rail Corridor Life‐Cycle Assessment (LCA) Framework, Factors and Models
Funding Sources: Federal Railroad Administration (FRA); project led by Michigan Technological University
The purpose of this three-year project is to establish a decision support framework for life cycle assessment (LCA) of emissions associated with railway track infrastructure maintenance. The framework will use life cycle assessment to quantify the greenhouse gas (GHG) emissions of manufacturing, installing and maintaining track components and infrastructure materials, including the emissions of machinery and processes required for maintenance of freight and passenger rail corridors in the North American context

–
Study of Spatial Trends in Freight Rail Traffic
Funding Sources: Railway Industry Supplier
This objective of this project is to predict future trends in traffic flows on the North American freight railroad network utilizing various statistical and Machine Learning methods. Phase 1 include collecting data that potentially can be used for traffic prediction; investigating relationships between various variables and rail traffic utilizing variable selection methods and regression models to demonstrate the relationships with traffic volume. Phase 2 includes constructing predictive models to achieve high accuracy using machine learning models featuring neural network methods.
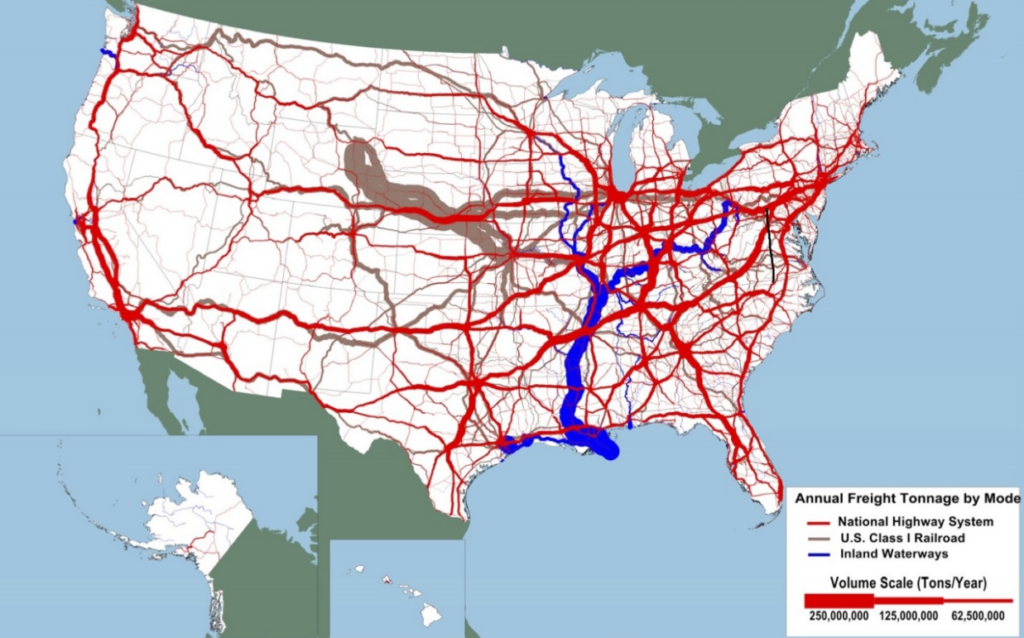