
Abstract— Big data and its production, management, and utilization are essential components in smart city planning. This paper presents a research framework for applying machine learning and deep learning using multiple big data sets on real estate. We built ensemble machine learning models to track property ownership variance in Austin, TX, USA. Then, the study employed the long short term memory (LSTM) model as a deep learning approach to forecasting property value in the same area. For model validation, root mean squared errors were calculated in both models. To
avoid underfitting or overfitting of LSTM, we experimented with specific parameters settings. Bagging-based random forest machine learning model outperformed other ensemble machine learning models. Regarding property ownership variance, the Random Forest model’s highest feature importance generally comprised the race, age of residents, land use and built environment factors, number of schools, and neighborhood location. Our LSTM model predicted Austin to retain a rising curve in housing prices and identified which part of Austin experiences an increase or decrease in property value. The predictive models may help city planners to quantify and gain insights on future impacts of developing neighborhoods.
avoid underfitting or overfitting of LSTM, we experimented with specific parameters settings. Bagging-based random forest machine learning model outperformed other ensemble machine learning models. Regarding property ownership variance, the Random Forest model’s highest feature importance generally comprised the race, age of residents, land use and built environment factors, number of schools, and neighborhood location. Our LSTM model predicted Austin to retain a rising curve in housing prices and identified which part of Austin experiences an increase or decrease in property value. The predictive models may help city planners to quantify and gain insights on future impacts of developing neighborhoods.
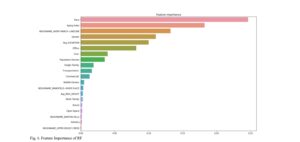
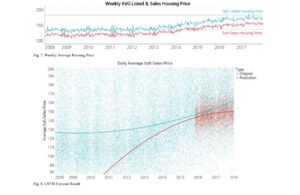
For more information, please visit: https://ieeexplore.ieee.org/document/9671298