Haijing Liu
Abstract
This paper studies the clustering effect of innovation spaces and its social implications in Shanghai. Using two kinds of spatial density analysis methods, the study first identifies the hotspot of innovation spaces, and then uses a statistical model to study the mechanism behind the clustering. The model demonstrates that rental housing units, IT companies, universities, restaurants, bars, and coffee shops have a positive impact, while large housing developments and parks have a negative impact on the clustering. The rapid establishments of innovation spaces also present a shift of urban development models in Chinese cities. Unlike the extensive urban developments that happened in the last decade, people and business value the more fine-grained urban developments that tie the social aspect of urban life back. The existence of innovation spaces not only generates an innovation network that facilitates innovation and entrepreneurship but also social interactions and a collective lifestyle.
1. Introduction
China responded to its economic deceleration, marked by an annual GDP growth rate dipping below 10% since 2011, with a national initiative promoting mass innovation and entrepreneurship. Scholars like Birch, (1981) and Baumol (2008) attribute significant job increases and successful urban growth to regions with the highest rates of innovation. They argue that entrepreneurship stimulates economic growth by applying innovative approaches in a competitive market.
Inspired by these insights, the Prime Minister launched a national initiative promoting “mass innovation and entrepreneurship” at the Summer 2014 Davos Forum. Soon afterward, during a State Council executive meeting in January 2015, the term “Collective Innovation Spaces” (CIS) emerged. CIS refers to physical platforms like coworking spaces, makerspaces, hackerspaces, and innovation centers, designed to support and facilitate innovation and entrepreneurial activities (Deng et al., 2020).
The central government’s policy suggestions in China significantly shape local governments’ decisions and the direction of the capital market. As a result, the number of CIS in China skyrocketed from just 50 before 2015, to 2300 in 2015, and further to 4000 in 2016 (Fig.1). This rapid growth has influenced many urban design projects and master plans, positioning CIS as a cornerstone of their design strategy.
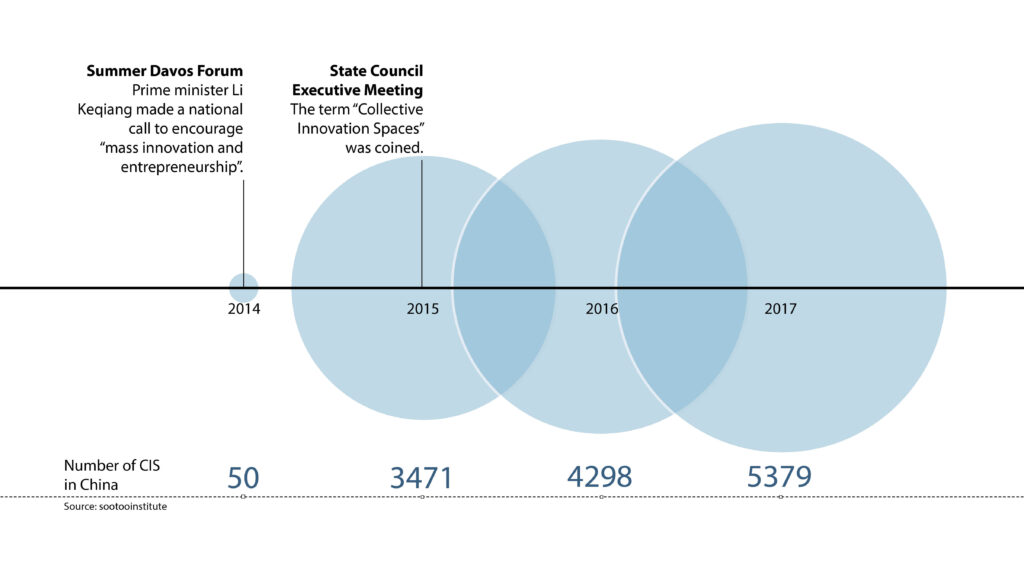
Previous studies on CIS have mostly approached the topic from a business and management perspective, seldom connecting it to its urban environment. This study offers a fresh perspective by concentrating on the role of urban amenities in CIS development, aiming to inform neighborhood scale planning and design decisions. Through interviews, surveys, and statistical spatial modeling, this research uncovers the significance of a balanced mix of affordable housing, workspaces, and social spaces in CIS clustering in Shanghai.
The study finds that established IT firms are the strongest predictors of CIS clustering. Accessibility to social spaces such as bars, restaurants, and coffee shops takes the second spot. The availability of rental units, which offer a more affordable housing option in China, correlates positively with the clustering of CIS. On the other hand, the presence of parks correlates negatively with CIS clusters.
These statistical findings suggest a shift in urban development models in Chinese cities. Unlike the extensive urban developments of the past decade, current trends show individuals and businesses leaning towards dense and mixed-use urban developments. These developments weave the social aspect of urban life back into the urban environment. CIS does more than create a network facilitating social interactions, innovation, and entrepreneurship—it revives the collective working and living style prevalent before the open reform.
In the subsequent sections, this study will delve into the theoretical background and conceptual framework, the data and methods, and the results and discussion, before offering a conclusion.
2. Literature Review
2.1. Why study CIS clusters?
New theories and ideas are increasingly examining the relationship between the characteristics of urban space and its impact on economic performance. In China, urban planning has frequently served as a tool for economic development. Spatial constructs such as science or high-tech parks, cultural districts, office parks, etc. have been framed as the spatial expression of the knowledge economy (Ali, 2013). CIS, as a rapidly growing market, not only offers physical infrastructure for local entrepreneurs, but also transforms the office landscape in the downtown area (Jamal, 2018).
The concept of the cluster originates from the field of economic geography, where Porter (1998) defined the clusters as “a critical mass” of collocated companies and institutions. In Porter’s theory, he identifies the locational competitive advantages fueling the clustering of industrial activities. Firms are inclined to seek proximity to an established industrial cluster to leverage the existing infrastructure, human capital, knowledge spillover, and input and output. While Porter emphasizes that collocated industries should be from the same category, Jacobs (1969) believes that diversity and the interdisciplinary nature of different sectors stimulate the emergence of new industries, thereby promoting a sustainable economic environment.. Glaeser et al. (1992) examined these theories and argued that a diversity of industries, rather than a monopoly, encourages knowledge spillover, expediting industry growth and employment increase. Although their industrial cluster analysis is at a regional scale, the clustering of CIS aligns with their theory at a smaller scale. CIS clusters can be seen as a mix of diversity and uniqueness. Few CIS has restrictions on the industry of the companies seeking entry, making these spaces a place for interdisciplinary knowledge exchange and collaboration.
On the other hand, there is a general spatial pattern of the industries within a city. For example, in Shanghai, IT companies tend to locate outside the downtown area, where new urban development takes place; design and advertising companies tend to locate inside the downtown area, where there are historical architectures and rich contexts. Thus, from the theoretical perspective, CIS clusters enable industrial clusters with diversity.
Furthermore, the study of CIS clusters, rather than isolated CIS, is motivated by the social network factor. The existence and sense of community is the core of collective innovation spaces, with the value of these communities residing within social networks. Activities such as social events, lectures, and pitches unfold in areas such as the lounge or the common area inside CIS, serving as platform and hubs for social interactions, innovation, and entrepreneurship. These platforms and hubs become connection nodes in the innovation network, making spatial proximity critical for the network’s viability. The more spaces a CIS is proximal to, the larger the network it resides in, enhancing the potential for users in these spaces to excel and innovate. Therefore, we anticipate that CIS clusters will foster economic prosperity.
2.2. Why place matters?
CIS clusters can illuminate the location choices of emerging firms. Audretsch and Feldman (1996) determined that innovative industries have a shorter cluster radius. Many scholars emphasize the importance of face-to-face interactions in productivity, asserting that locations facilitating “close contact and exchanging ideas” generate economic growth and social values more efficiently (Feldman & Choi, 2015; Glaeser et al., 2010). These scholars focus on a more granular scale than traditional industrial cluster literature, centering on quality of life and urban ammenties that attract skilled workers rather than solely on the business interest of firms.
Florida (2008) argues that firms relocate with human capital, underscoring the importance of creating the desired quality of place that attract human capital. This argument inspired many scholars to outline a range of urban amenities that appeal to knowledge workers and the creative class (Chatterji et al., 2014; Florida, 2002).
However, the relationship between firms and individulas is complex. People might choose to move to a particular city primarily due to abundant job opportunities, with urban amenities serving as secondary incentives influencing their preferences for a specific location within the city (Darchen & Tremblay, 2010). Therefore, I designed this study to focus in one particular city, Shanghai, where the neighborhood scale provides a more suitable lens for examining how the quality of space and urban amenities influences the location choice of firms and individuals.
2.3. Theoretical Frameworks of Quality of Place
Extensive existing literature delves into how non-market public goods, such as affordable housing, transportation, healthcare, education, leisure facilities, retail, and natural amenities render certain places more attractive to the creative class and knowledge workers (Florida, 2002, 2008; Glaeser et al., 2001; Insch & Florek, 2008; Kunzmann, 2012; Yigitcanlar et al., 2007).
Although many scholars have emphasized the importance of specific urban amenities to the creative industry and entrepreneurship, few empirical studies have tested these theories in Chinese cities. Simultaneously, an increasing number of cities are adopting the concepts of innovation districts or knowledge-based urban development for urban redevelopment or new town planning projects. Without an accurate understanding of the mechanism underlying the innovation ecosystem, these concepts merely serve as buzzwords to attract the support of the central government and brand projects for mass media consumption. Thus, this paper is one of the first attempts to illuminate the design and planning of knowledge-based urban development in China.
Most literature is grounded in the context of the U.S. and other Western countries and CIS, specifically coworking spaces are argued to have a strong influence on downtown redevelopment (Jamal, 2018). Taking into account the cultural differences between China and Western countries, this paper revisits recent urban development models in China, which are tied closely to the nation’s economic and political agenda. The context provided by these recent three development models aids in constructing this paper’s analytical framework.
2.3.1. Danwei
The “danwei” represented the “basic unit of urban life” during the central planning period in socialist China. As Bray defines it (Bray, 2005), “danwei is a generic term denoting the Chinese socialist workplace and the specific range of practices that it embodies, which marks a common system shared by all urban Chinese workplaces.” A typical danwei model is architecturally designed as a walled or fenced compound with controlled access points (gates). A danwei complex integrates three types of spaces: spaces for living, spaces for working and spaces for social services. While these spaces have distinct functions, they remain physically interconnected or juxtaposed (Fig. 2).
In general, the danwei merges life, work and play within a singular city unit. Through spatial design, it fosters robust cohesion within the commune, seamlessly blending life and work into a unified existence.
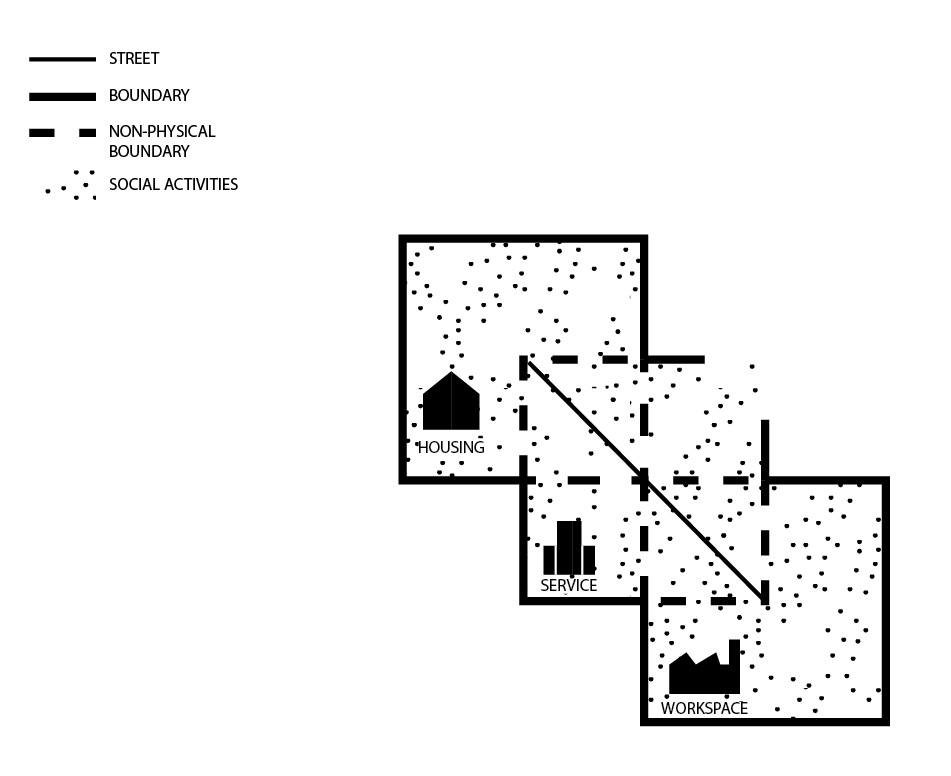
2.3.2. High-tech Development Zone
Following the open door policy of 1978, China concentrated its efforts on revitalizing and reforming science and technology development. In 1988, the Torch Program was initiated to accelerate this sector’s development. Adhering to the theory of industrial agglomeration and taking inspiration from models like Silicon Valley and Route 128, high-tech development zones (HIDZs) were introduced in urban peripheries where large green land parcels were available. Its design prioritizes car usage, which are featured by large blocks and wide roads. Single-use zoning is employed to facilitate the agglomeration of high-tech research and production. Most of HIDZ were located on farmlands isolated from the urban center. Incubators, as an early form of CIS, were mainly located in HIDZ or industrial parks.
The spatial layout of HIDZ represents a radical departure from traditional Chinese city layouts or the danwei configuration. Streetscapes, once vibrant arenas for daily social interactions, lost their distinctive socializing characteristics. A clear segregation emerged between living spaces, workspaces, and public spaces, becoming the primary flaws of HIDZs. “Big-box” shopping malls were introduced as a substitute for social spaces. However, unlike danweis or traditional urban spaces-where an intimate building scale facilitates encounters and social interactions – the HIDZ’s built environment inhibits everyday social activities due to reduced foot traffic and difficult access to social spaces.
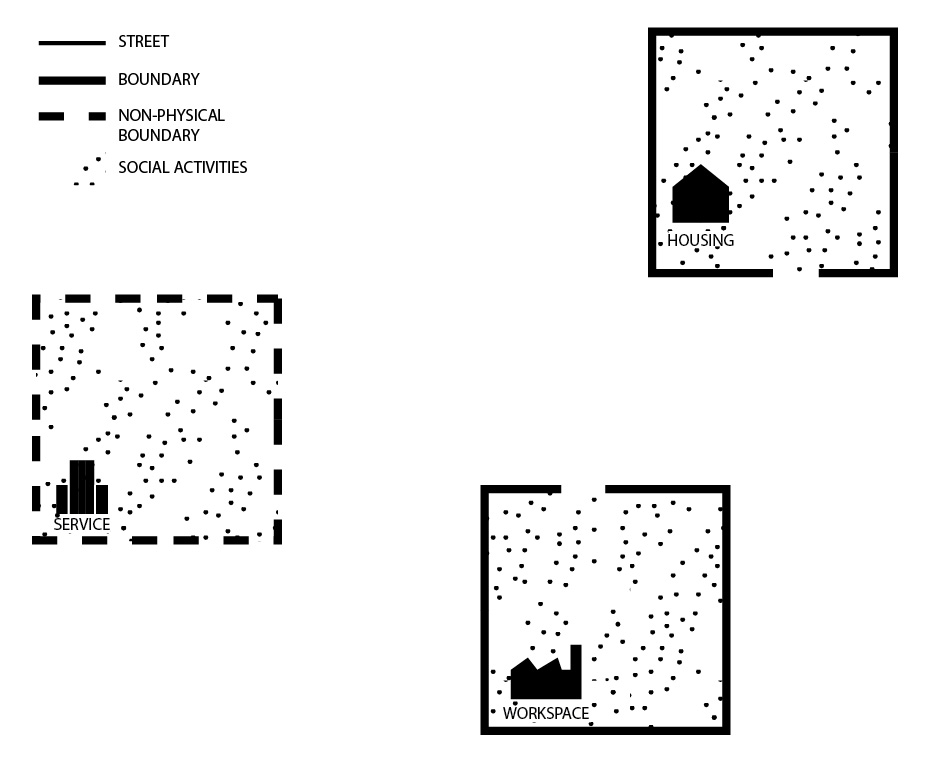
2.3.3. Knowledge-based Urban Development
Knowledge-based urban development (KBUD), also known as innovation districts, has emerged as a primary economic strategy for numerous city governments. While the Chinese government originally advocated for CIS rather than innovation districts, many recent master plans have emerged the buzzword “innovation and entrepreneurship”. Diverging from previous urban typologies, KBUD places a greater emphasis on characteristics centered around individuals. Two key elements are the quality of urban life, and the availability of social spaces for interaction.
According to Katz and Wagner (Katz & Wagner, 2014), the critical elements of innovation districts include integration with their urban context, disruption of organizational hierarchies, fostering interactions and collaboration between industries and other knowledge communities, encouraging face-to-face interactions and ultimately promoting technological advancement.
As describes, “Knowledge community precincts in a city are not ivory towers in the urban jungle, nor communities gated against visitors and burglars. They are, ideally, catalytic locations for urban life. They are experimental life spaces for the next urban generation and laboratories for testing new forms of work-leisure-home lifestyles.”
From a design perspective, KBUD necessitates a compact spatial layout to increase density; integration with existing urban fabric; connectivity to public transportation; a diversity of programs and functions as well as a diverse population. Further, it needs to promote walkability and bikability, and provide public spaces and social areas for interaction, communication and collaboration (Katz & Wagner, 2014; Kunzmann, 2012; Yigitcanlar, 2010).
The concept of “third places”, coined by sociologist Ray Oldenburg, refers to those locations where people spend time apart from home (first place) and work (second place). These areas, including cafes, parks, and communal spaces, provide settings for idea exchange, relationship-building, and broader creative interactions. As such, the advent of CIS developments in Chinese cities could reestablish the proximity between workspaces, living spaces, and these crucial third places featuring what Yigitcanlar (2010) describes as “the new forms of work-leisure-home lifestyles”. Through the network constructed by CIS and other types of third places, spaces for social interactions and the sense of community will also resume in everyday life in Chinese cities.
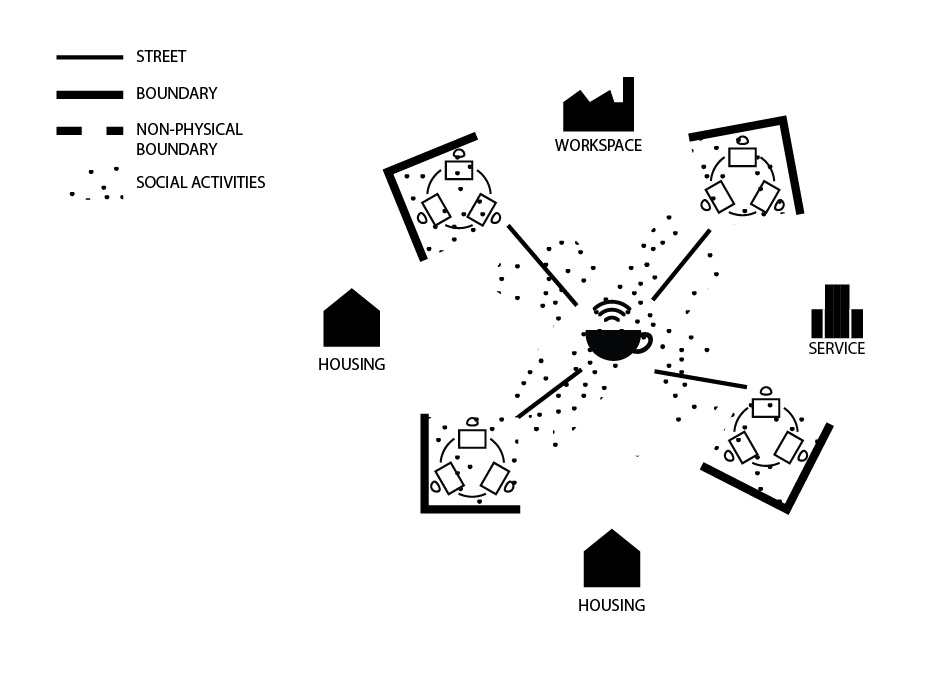
To conclude, as we delve into the analysis of urban development models in China, it’s crucial to acknowledge that while many scholars have pointed out the importance of specific urban amenities to the creative industry and entrepreneurship, very few empirical studies have been conducted in Chinese cities to investigate these theories. This gap in research becomes all the more significant as more cities globally, including those in China, are adopting the concept of innovation districts or knowledge-based urban development for their urban redevelopment or new town planning projects.
However, without a precise understanding of the mechanisms underlying the innovation ecosystem, the popular terminologies associated with it often end up being used merely as titles to garner central government support and to brand projects to the mass media. This reality underscores the need for in-depth research into the subject.
To this end, this paper serves as one of the pioneering efforts to draw conclusions from both existing literature and current practices in CIS to illuminate the design and planning of knowledge-based urban development in China. Through the exploration of urban amenities that are crucial to CIS clusters, I seek to provide a more comprehensive perspective on the phenomenon and practical guidance for future developments.
3. Data and Methodology
The data collection of this study consists of two parts. The first part of the data collection is interviews with CIS users and managers. The result of this part, along with the literature review helps to establish the conceptual framework of the statistical model that examines the relationship between urban amenities and CIS clusters. The second part of the data collection is to construct the statistical model, which relies on multiple online sources to obtain the location of CIS and urban amenities.
3.1. Interview
At the beginning of 2018, the author went for a site visit to several CIS. Interviews were conducted with 18 space users and 15 space managers. Space users claim restaurants and convenient stores are the most visited among CIS users. 72.2% of the users claim to be frequent visitors to convenient stores and restaurants. 50% of the users claim to be frequent visitors to coffee shops. 11 out of 17 users live in rental units, and their primary transportation method is by metro. Here (Figure 4) is a summary of the interview results, which will help construct the conceptual framework in the next chapter.
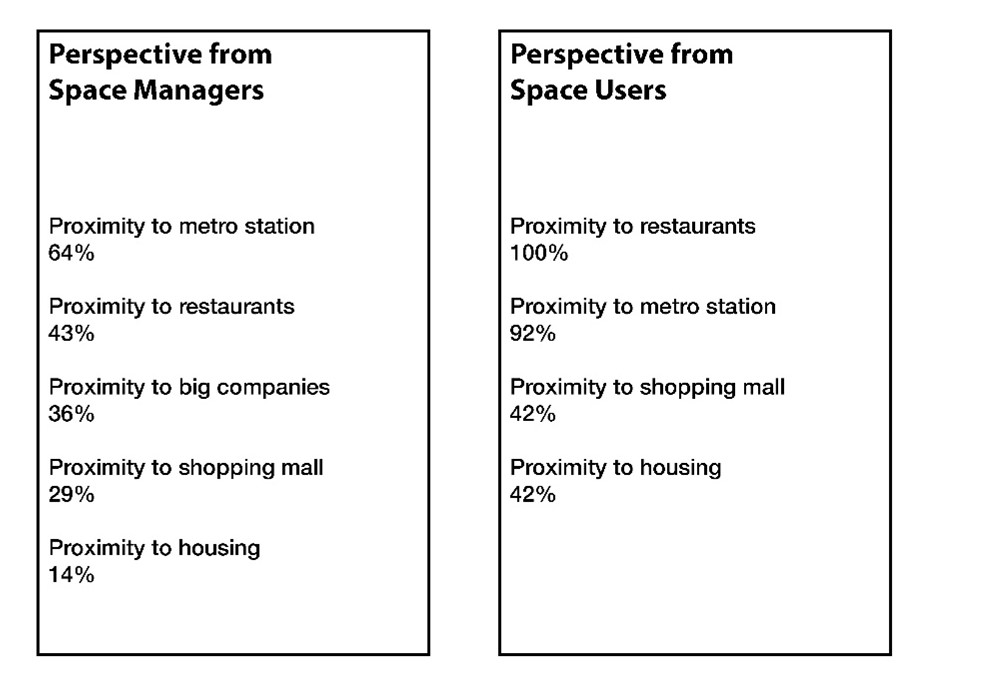
3.2. The Statistical Model
3.2.1. Data
Data for the statistical model was primarily sourced from multiple online sources. The primary dataset for CIS was scraped from Ctoutiao in 2018, comprising 716 entries with name and address. However, among these 716 entries, some were missing while some others did not meet the CIS definition utilized in this study. Consequently, the author initiated a four-step verification process to refine the dataset.
Firstly, entries such as high-tech development zones, tech-parks, creative industrial clusters, and office parks were removed because they do not conform to this paper’s definition of CIS. Secondly, the author conducted site visits to confirm that locations listed as CIS on the website operate as CIS according to the central government’s definition. As such, observations that contradicted this were removed.
In the third step, the author noticed missing locations from specific CIS chain brands, like People Square and FT Town, among others. To fill these gaps, the author visited the respective brand websites and scraped all the location data to add to the list. Lastly, the list did not include entries from international coworking brands such as WeWork. These were subsequently added to the dataset. The final list consisted of 361 collective innovation spaces, complete with names, geolocations, and brand names.
The UDparty online data platform, which provides access to rental and POI data in Shanghai, was also utilized. The rental data includes street address, floor area and price. The POI data has address and categories.
3.2.2. Model Specification
In this model, the dependent variable measures the accessibility of a given CIS to other CIS within a walking distance. The higher the accessibility to other CIS within walking distance, the more likely that the CIS is located within a cluster that promotes regular face-to-face interactions crucial for stimulating innovation. Most literature regards 1000m as a standard threshold for walking distance.
The independent variables have been chosen following the theoretical framework of live, work and play. For living spaces, the indicators include accessibility to housing and rental units. The working spaces are gauged by accessibility to anchor companies (as per the POI dataset), IT companies, distance to the nearest university, and accessibility to research institutes. Social spaces are evaluated based on the accessibility to coffee shops, bars, parks, Chinese and exotic restaurants. Control variables include accessibility to restaurants, entertainment facilities, metro stations, retail and distance to the city center[1].
The gravity index is used to measure accessibility.

represents a node i at a radius of r is defined as follow (Sevtsuk & Mekonnen, 2012):
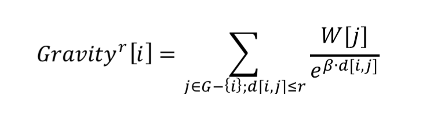
(2)
ℬ is the exponent that controls the effect of distance decay on each shortest path between i and j, d[i,j] is the distance between i and j. In this paper, ℬ is set to be 0.002 following (Sevtsuk, 2017). W[j] is the weight of a specific destination j that is within the defined radius r from i. Due to data limitations, this paper will not consider any weights for the destinations.
[1] People Plaza as the city center.
4. Results and Discussion

From Fig. 6, it’s evident that CIS clusters are primarily located in downtown areas, certain high tech parks, and some recently developed knowledge-base innovation districts. Although the map provides a broad spatial pattern of these places, the statistical model will offer a more in-depth understanding of the mechanisms underlying CIS clustering.
The dependent variable of table 2 is the gravity index of CIS, which indicates its degree of clustering. Table 2 presents four models: live, work, play and live-work-play. The live model only includes parameters associated with living spaces and control variables. The work model only includes parameters associated with workspaces and control variables. The play model only includes parameters associated with social spaces and control variables. The live-work-play model includes all parameters and control variables.
The adjusted r-square value in the first three models reveals that the work model consistently has the highest predictive power on CIS clusters. This result implies that industry influences CIS clusters more significantly than the other two factors. This finding is consistent with the literature (Darchen & Tremblay, 2010; Storper & Scott, 2009) that career choices among knowledge workers/ or the creative class have a greater influence than the quality of space. The adjusted r-square of the live-work-play model is higher than the work model. This indicates although industry foundation plays a vital role in the clustering of CIS, the quality of space also has a positive effect. The following section will examine each parameter in the model in more detail.
4.1. Industry Foundation & Agglomeration Effect – the Work Model
In terms of industry, two factors significantly contribute to the clustering of CIS. When accessibility to IT firms increases by one unit, the gravity index of the CIS increases by 0.162. When the distance to the nearest university decreases by one kilometer, the gravity index of the CIS increases by 0.4. These results indicate that CIS tend to cluster near IT companies and universities. While anchor companies and research institutions are often considered as important institutions for industrial clusters in literature, they do not have a notable impact on the clustering of CIS.
The effect of universities on innovation industries is well documented(Chatterji et al., 2014; Pittaway et al., 2020). A prevalent understanding is the potential for innovation spillover from universities. Being near a university means more cooperation with professors and research labs, expediting the commercialization of innovation. Another potential factor driving CIS clustering around universities comes from the policy side. The mass innovation and entrepreneurship initiative encourage college graduates to start their own companies. College students are potential users for CIS, and many CIS are jointly funded by universities and government, meaning universities are likely to provide space for CIS. Moreover, being near universities means affordability and accessibility to knowledge for CIS users. For instance, one CIS user stated the advantages of being close to universities include access to affordable meals in the university cafeteria the opportunity to attend lectures and talks hosted by the university, which are especially beneficial for early entrepreneurs and high-skilled workers.
Despite the influence of the IT industry being seldom documented in the literature, accessibility to IT companies remained strongly significant in all robutness checks, and had a relatively high coefficient. As in many news articles, The rapid expansion of the IT industry in China in recent years has been a phenomenon recorded (Wang & Loo, 2017). The IT industry’s growth rate surpasses other industries and has generated a large number of startups, such as platforms related to the shared economy, e-commerce, and also new media (The Economics, 2018). Therefore, IT startups are considered as the major users of CIS. CIS tend to cluster in an area where it has a high density of IT companies in order to gain access to a larger pool of potential users.
4.2. Affordable Living Options – the Live Model
Regarding living spaces, accessibility to rental units has a positive effect on the clustering of CIS. This statistical finding aligns with the interview data, which shows 64.7% CIS users are renters. Results from Table 2 suggest that when the gravity index between CIS and rental units increases by one, the gravity index of CIS will increase by 0.041. These empirical results suggest that CIS tend to cluster in places where there are more available rental units.
One explanation for this is that many CIS users are recent college who cannot afford to purchase housing units in Shanghai. Another explanation is that many entrepreneurs consider their time to be extremely valuable. Given the intense market competition, many entrepreneurs do not have regular working hours or weekends off. Consequently, entrepreneurs cannot afford long commuting hours and being able to live nearby is critically important.
It should be noted that there is a limitation in the data due to the lack of inclusion of the subway network in the calculation. As the interviews indicate that 70.6% of CIS users commute by metro, when calculating the gravity index between CIS and housing units, the subway network should ideally be included in the calculation. However, due to limitation in the subway network data, this paper only considers the accessible rental units within the 1000m walking radius.
4.3. Social Spaces
For social spaces, the accessibility to coffee shops, bars, and Chinese restaurants positively influences the clustering of CIS, while parks seem to have a negative effect. While findings for coffee shops and restaurants are in line with existing literature (Green, 2014; Jackson, 2017; van Oort et al., 2003), the impact of parks contradicts one paper by Yigitcanlar (Yigitcanlar et al., 2007). There are three potential explanations for this discrepancy.
First, Yigitcanlar’s study focused on Australian cities, where have a different cultural context. Second, many parks in Shanghai are large, often isolated by major infrastructures, such as highways, resulting in lowperceived accessibility. Lastly, office buildings near parks typically come at a higher cost. Startups or CIS users are less likely to pay a premium for proximity to parks or open spaces, leading to a lack of CIS clustering around these areas.
In China, restaurants often serve as primary locations for meetings and business interactions, making them important social space for entrepreneurs. Unlike parks, restaurants, bars and coffee shops are privately owned public spaces. Among these semi-public spaces, bars have the highest coeffient, suggesting they play a significant role in social interaction. Bars provide a less formal setting than resturants. In Chinese culture, dinning in a restaurant comes with many unspoken rules regarding seating and drinking, whereas bars do not typically have such rulesand are favoured by young people for their vibrant and relaxed atmosphere.
The model highlights the increasing importance of various types of social spaces. They offer the quality of space favoured by knowledge workers and the creative class. Therefore, CIS tend to cluster in places that strike a good balance between social space, work opportunities, and affordable housing.
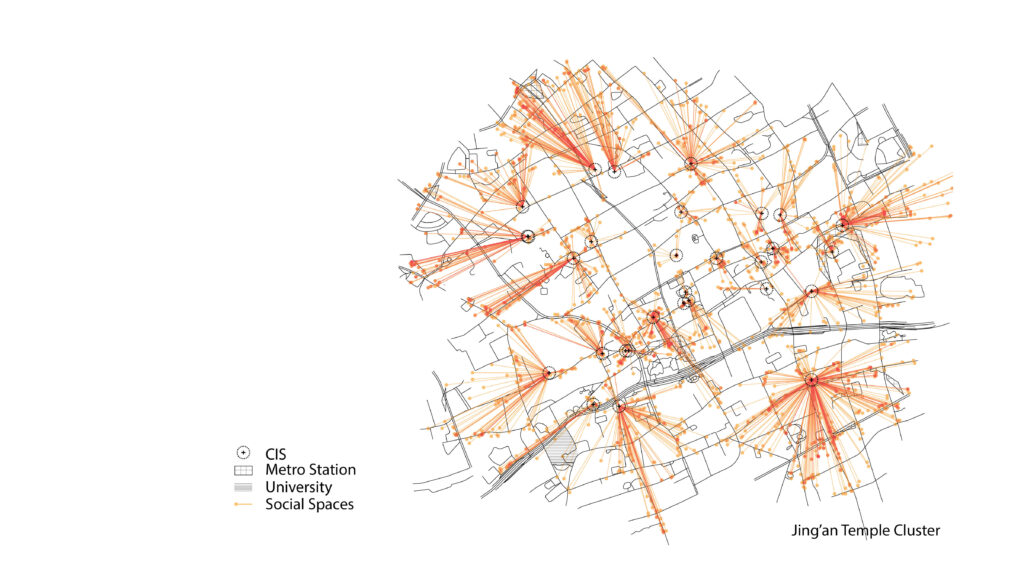
4.4. Discussion
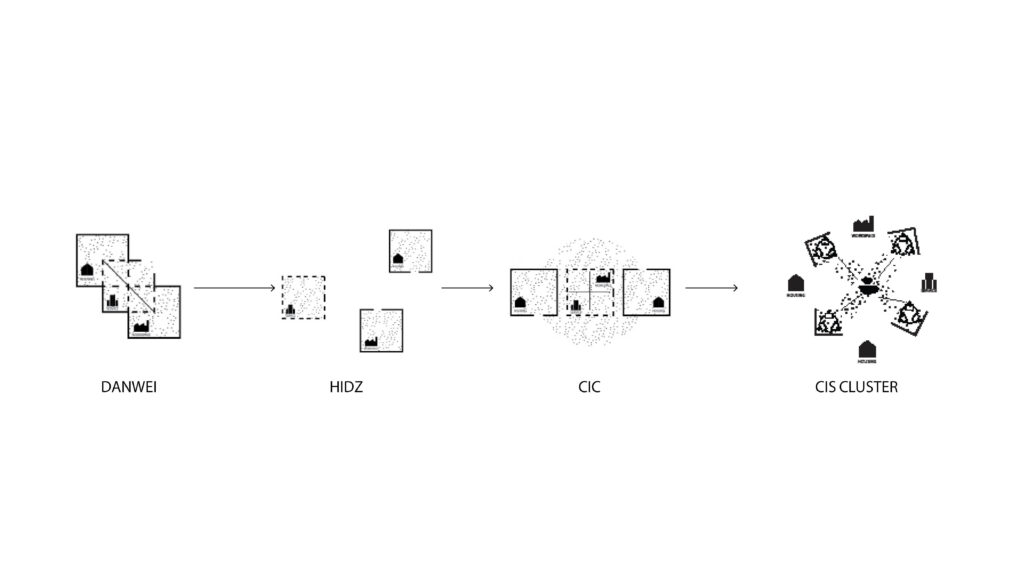
The previous section took a retrospective look at different urban development models across varying time points to understand the Chinese context for constructing the statistical model. Now, I want to revisit these urban development models with a different focus, especially on their planning process and social implications.
Urban development models aren’t merely tools for city growth and economic advancement. They are also social constructs that reflect society’s values, priorities, and aspirations. The planning process, as well as the resulting urban environment, can shape societal structures, cultural values, and quality of life.
The danwei system, as described above, can be seen as an intricate social and physical unit that includes both the residential and work elements. It’s a concept that integrates many facets of life – work, social interactions, and all aspects of living – within one structure. People in this system are colleagues, neighbors, and friends, creating a multifaceted network of relationships.
The structure of a danwei, as characterized by Bjorklund, is essentially multifunctional, where workspaces can morph into social spaces, and household events can spill over into common areas (Bjorklund, 1986). There’s no stark delineation between different types of activities; instead, all aspects of life seamlessly blend and interact with each other. This gives rise to a uniquely intertwined socio-spatial environment where social interactions often occur in transitional spaces such as roads, alleys, or hallways.
The danwei design also fosters a strong sense of community. Its intimate scale and gated complex create a shared identity and communal bond among its residents. However, this shared identity can also work as a double-edged sword, as the physical and administrative barriers of a danwei can isolate its community from the rest of the city, potentially limiting exposure to diverse social and cultural experiences.
Although the planning of HIDZ have guidelines for allotting public spaces such as parks, community centers, recreation centers, the accessibility of these spaces can often be a challenge. Planning regulations might stipulate a certain number of these public facilities per population count; however, this does not necessarily translate to their effective use. .
The issue of accessibility arises when these public spaces are situated on large parcels of land, often bounded by expansive road networks, which effectively means they serve a much larger catchment area. This leads to people residing in HIDZs often needing to drive considerable distances to access these spaces, which should ideally be close to their homes and easily accessible on foot or by public transit. This inaccessibility tends to inhibit regular use of these spaces, consequently stifling opportunities for social interactions and community-building.
In essence, the placement and distribution of public spaces in HIDZs can often result in spatial segregation, reducing the sense of community among residents. It also highlights a common urban planning challenge: how to design and implement public spaces that not only meet quantitative criteria, but are also readily accessible, encourage social interactions, and enhance community cohesion.
Historically, urban planning in China heavily relied on metrics, with the focus primarily on meeting the basic human needs of everyday life. More recent practices, however, have adopted more comprehensive and fine-grained approaches. CIS tend to cluster in areas where there is a comprehensive ecosystem in place. They can succeed in various ways through a sophisticated approach, as opposed to following a simple checklist.
One of the unique aspects of the CIS development model lies not only in its fine-grained scale, allowing for a more organic planning approach, but also in its nested social space that helps foster a sense of community among various groups of people. During my visits to several CIS in Shanghai, I observed that different spaces within the CIS serve different social functions. The lounge areas are generally accessible to the public and often host events such as pitches, demo days, and lunch lectures with entrepreneurs and venture capitalists. I noted during my weekday afternoon visits to People Squared and Kr space that the lounge area was bustling with people meeting in various groups. The unique design of the public space in places like Mixspace also attracts visitors. Moreover, many CIS provide not only lounge areas but also more private common areas exclusive to their users, offering a peaceful space for rest and reflection.
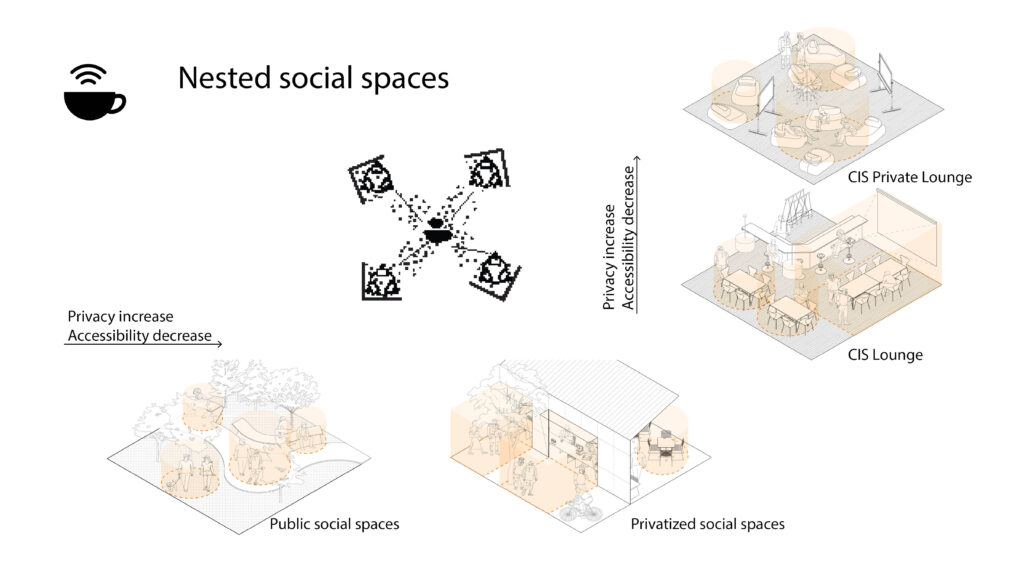
At the neighborhood scale, private domains such as restaurants, coffee shops, and bars complement the CIS, creating nested social spaces with varying levels of privacy and accessibility (See Fig.9). These nested social spaces foster community formation, adding another layer of vibrancy to these urban areas.
5. Conclusion
This study explores the location preferences of CIS in Shanghai, focusing on how these decisions impact social spaces and urban living. The statistical model used in this research offers valuable insights into the decision-making process of CIS developers and their motivations for clustering in certain areas. Based on the theory that CIS developers select locations based on nearby amenities that appeal to their primary users—startups and young entrepreneurs—the model’s results largely corroborate the existing literature, confirming that factors like industrial heritage, social interaction spaces, and proximity to universities positively impact CIS clustering (Audretsch & Feldman, 1996; Florida, 2002; Glaeser et al., 2001).
However, the model also reveals some inconsistencies with existing theories. For instance, the negative correlation between the presence of parks and open spaces and CIS clustering contradicts Yigitcanlar’s argument about the importance of outdoor activities to young entrepreneurs (Yigitcanlar et al., 2007). This discrepancy opens a new avenue for further investigation.
Unique to Shanghai’s context, the model reveals a strong correlation between the presence of IT companies and CIS clustering. Additionally, the model shows that increased access to rental units positively impacts CIS clustering, offering a new insight into the locational preferences of CIS developments in Shanghai. Complementing the statistical model, this paper also incorporates data from interviews, surveys, observations, and mappings to more thoroughly understand the social implications of CIS clusters. The CIS model, integrated with ‘third places’ like coffee shops and restaurants, encourages a renewed sense of community and public engagement. It blends with the urban fabric, dissolves boundaries, fosters networks, and stimulates diversity and conversations among various social groups. This integrated, multidisciplinary approach, combining quantitative modelling with qualitative analysis, allows for a more nuanced understanding of how CIS developments influence social dynamics and urban living in Shanghai.
6. References
Ali, M. (2013). Knowledge economy and the city: Spaces of knowledge. In Knowledge Economy and the City: Spaces of Knowledge. Routledge. https://doi.org/10.4324/9780203816141
Audretsch, D. B., & Feldman, M. P. (1996). R & D Spillovers and the Geography of Innovation and Production. The American Econnomic Review, 86(3), 630–640. https://doi.org/Article
Baumol, W. J. (2008). Entrepreneurs, Inventors & the Growth of the Economy. The Conference Board EPWP, 1–15. https://hcexchange.conferenceboard.org/pdf_free/workingpapers/E-0039-08-WP.pdf
Birch, D. L. (1981). Who creates job? The Public Interest, 65(Fall), 3–14.
Bjorklund, E. M. (1986). The Danwei : Socio-Spatial Characteristics of Work Units in China ’ s Urban Society. Economic Geography, 62(1), 19–29. https://doi.org/10.2307/143493
Bray, D. (2005). Social Space and Governance in Urban China. In Stanford University Press. https://doi.org/10.2307/20066144
Chatterji, A., Glaeser, E., & Kerr, W. (2014). Clusters of Entrepreneurship and Innovation. Innovation Policy and the Economy, 14(1), 129–166. https://doi.org/10.1086/674023
Darchen, S., & Tremblay, D. G. (2010). What attracts and retains knowledge workers/students: The quality of place or career opportunities? The cases of Montreal and Ottawa. Cities, 27(4), 225–233. https://doi.org/10.1016/j.cities.2009.12.009
Deng, K., Ding, Z., & Xu, M. (2020). Population agglomeration and the effectiveness of enterprise subsidies: a Chinese analysis. Regional Studies, 54(8), 1136–1148. https://doi.org/10.1080/00343404.2019.1681586
Feldman, M. P., & Choi, J. (2015). Harnessing the Geography of Innovation. In The Handbook of Global Science, Technology, and Innovation (pp. 267–289). John Wiley & Sons, Ltd. https://doi.org/10.1002/9781118739044.ch13
Florida, R. (2002). The Rise of the Creative Class: The New Global Competition for Talent. In The Rise of the Creative Class. HarperBusiness.
Florida, R. (2008). The Economic Geography of Innovation. 92(4), 808–882.
Glaeser, E. L., Kallal, H. D., Scheinkman, J. A., Shleifer, A., Scheinkman, J. A., Barro, R., Becker, G., Heim, C., Henderson, V., Katz, L., Lucas, R., Rosen, S., Rotemberg, J., & Schultz, T. W. (1992). Growth in Cities. Source Journal of Political EconomyJournal of Political Economy, 100100177(6). http://www.jstor.org/stable/2138829
Glaeser, E. L., Kerr, W. R., & Ponzetto, G. A. M. (2010). Clusters of entrepreneurship. Journal of Urban Economics, 67(1), 150–168. https://doi.org/10.1016/j.jue.2009.09.008
Glaeser, E. L., Kolko, J., & Saiz, A. (2001). Consumer city. Journal of Economic Geography, 1(1), 27–50. https://doi.org/10.1093/jeg/1.1.27
Green, R. (2014). Collaborate or Compete: How Do Landlords Respond to the Rise in Coworking? Cornell Real Estate Review, 12, 52–59.
Insch, A., & Florek, M. (2008). A great place to live, work and play. Journal of Place Management and Development, 1(2), 138–149. https://doi.org/10.1108/17538330810889970
Jackson, L. (2017). The importance of social interaction in the co-‐working spaces of Boston USA and London UK. European Media Managers Association Conference.
Jacobs, J. (1969). The Economy of Cities New York. JacobsThe Economy of Cities1969. http://www.bodysportsboutique.com/table-of-contents-the-economy-of.pdf
Jamal, A. C. (2018). Coworking spaces in mid-sized cities: A partner in downtown economic development. Environment and Planning A, 50(4), 773–788. https://doi.org/10.1177/0308518X18760857
Katz, B., & Wagner, J. (2014). The Rise of Innovation Districts : A New Geography of Innovation in America. Brookings Institution, May, 1–34. http://www.brookings.edu/~/media/Programs/metro/Images/Innovation/InnovationDistricts1.pdf
Kunzmann, K. R. (2012). The Strategic Dimensions of Knowledge industries in Urban Development The Strategic Dimensions of Knowledge Industries in Urban Development. 3625. https://doi.org/10.1080/02513625.2009.10557034
Pittaway, L., Aissaoui, R., Ferrier, M., & Mass, P. (2020). University spaces for entrepreneurship: a process model. International Journal of Entrepreneurial Behaviour and Research, 26(5), 911–936. https://doi.org/10.1108/IJEBR-09-2018-0584/FULL/PDF
Porter, M. E. (1998). Clusters and the New Economics of Competition Harvard Business Review. Harvard Business Review, 77–90. https://doi.org/10.1042/BJ20111451
Sevtsuk, A. (2017). Integrating Urban Design and Analytics Andres Sevtsuk. 1–32.
Sevtsuk, A., & Mekonnen, M. (2012). Urban network analysis. A new toolbox for ArcGIS. Revue Internationale de Géomatique, 22(2), 287–305. https://doi.org/10.3166/rig.22.287-305
Storper, M., & Scott, A. J. (2009). Rethinking human capital, creativity and urban growth. Journal of Economic Geography, 9(2), 147–167. https://doi.org/10.1093/jeg/lbn052
van Oort, F., Weterings, A., & Verlinde, H. (2003). Residential amenities of knowledge workers and the location of ICT-FIrms in the Netherlands. Tijdschrift Voor Economische En Sociale Geografie, 94(4), 516–523. https://doi.org/10.1111/1467-9663.00278
Wang, B., & Loo, B. P. Y. (2017). Hubs of Internet Entrepreneurs: The Emergence of Co-working Offices in Shanghai, China. Journal of Urban TechnologyOnline) Journal China, Journal of Urban Technology, 243, 1063–1732. https://doi.org/10.1080/10630732.2017.1285124
Yigitcanlar, T. (2010). Making space and place for the knowledge economy: Knowledge-based development of Australian cities. European Planning Studies, 18(11), 1769–1786. https://doi.org/10.1080/09654313.2010.512163
Yigitcanlar, T., Baum, S., & Horton, S. (2007). Attracting and retaining knowledge workers in knowledge cities. Journal of Knowledge Management, 11(5), 6–17. https://doi.org/10.1108/13673270710819762
About Author
Haijing Liu is a Ph.D. candidate in Community and Regional Planning at the University of Texas at Austin. Her dissertation research employs a mixed-methods approach to unravel the dynamics of the U.S. green job market, focusing on its distribution and growth across metropolitan areas. Liu’s work sheds light on significant disparities within this sector, emphasizing the underrepresentation and wage inequities faced by African Americans, Latinos, and women. Her research, deeply rooted in the ‘three-E’ paradigm—environment, economy, and equity—provides valuable insights into the interplay between institutional capacities and local economic structures. This approach not only highlights disparities but also suggests pathways for more equitable and sustainable development. Beyond her dissertation, she is actively engaged in exploring wider economic development and sustainability issues, with a keen focus on addressing gender disparities in the green job market and examining the broader societal readiness for sustainability transitions.