Mickey Edwards
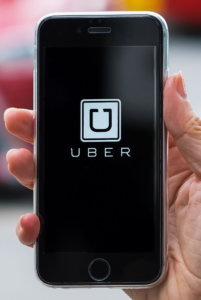
Abstract
This paper examines twelve UberX rides completed over four hours in Cincinnati with the intent of comparing first-person evidence of ridehail travelers to a growing body of quantitative literature. Traveler data are based on observation and casual conversation between the author (driver) and the passenger. A typical Saturday morning was chosen beginning in Cincinnati’s Central Business District (CBD), and each subsequent trip was based on the location of the previous trip destination without intervention. This work attempts to tell the narrative of where each traveler was going, infer why they chose ridehailing, and explore the social relationship between riders and drivers. More specifically, it places these twelve travelers in the context of published ridehail literature. From the driver’s perspective, $68.32 was grossed after four hours of driving—including one tip, $2 tip on the twelfth ride. This small sample of ridehail passengers, and driver profits, conforms to findings published in the academic literature yet is not intended to be statistically significant. This work has implications for future research by presenting details about trips and passengers not before seen in the literature.
Keywords
Ridehail; Uber; Socioeconomics; Driver Earnings
About the Author
Mickey Edwards, PhD, is a Visiting Researcher at the University of Illinois Springfield where he studies motor vehicle crashes and their effect on pedestrians, cyclists, and communities. Other research interests include transportation and infrastructure equity. He has worked on policy in the U.S. Senate and taught several courses at the University of Cincinnati. Previous careers include several years in photojournalism and a stint as an engineer for a large consumer goods company.
Introduction
Most of what researchers know about who uses ridehailing and why they use it is based on large, aggregated data sets. These data sets are certainly useful in modeling ridehail use and travel behavior, but the nuances of an individual’s options and reasons for transport choices are commonly lost. This paper sets out to explore a qualitative gap in the ridehail literature by presenting first-person, casual, statistically insignificant observations and conversations experienced during twelve UberX rides over 4 hours on a brisk March Saturday morning in Cincinnati, Ohio. The aim here is not to dispute nor support previous ridehail research, but to supplement it with descriptions of human behavior—too messy to quantify or model. Behavioral decisions made by both drivers and passengers are seemingly often influenced by how they perceive it will affect their star rating.
Ridehail companies depend upon user-facing performance ratings not only to monitor the behavior of their drivers, but also to monitor the behavior of their customers. Ratings for drivers and riders alike, though seemingly inconsequential at face value, can negatively affect either party when relatively low. Drivers face the threat of being de-platformed—essentially banned from driving for the company over a low average rating. Riders with a low average rating may face a relatively difficult time catching a ride—since some drivers are reluctant to pick up poorly rated passengers (Campbell, 2015). The social awkwardness that performance ratings sometimes hasten has not been lost on popular culture. Saturday Night Live has produced two sketches titled “Uber for Jen’’ and “Five Stars” (links provided in Reference section) with a combined YouTube audience of 14 million views satirizing the effect of star ratings on social behavior—specifically the social relationship between ridehail drivers and passengers. This research takes aim at that social relationship alongside glimpses into motivational factors for choosing ridehailing and the sociodemographic characteristics of riders.
Related Literature
Though some researchers have driven for Lyft and Uber in an effort to collect data, none have published findings collected in the same manner as presented here. Despite this, related literature is reviewed to provide context for passenger encounters described below.
Commonly Applied Methods and Sources
Ridehail researchers have used a variety of methods and data sources to answer a variety of questions. Rayle et al. (2015) intercepted and surveyed users observed exiting a ridehail vehicle at popular San Francisco destinations in their exploratory and oft-cited study. Henao (2017) drove for Lyft and Uber in Denver to overcome a dearth of available data and directly accessed 311 passengers for surveying. Gehrke et al. (2018) recruited 10 ridehail drivers in Boston to implement a passenger survey, paying them $2 for each of the 944 completed responses. Clewlow and Mishra (2017) emailed surveys to 4,094 respondents in 7 major American metropolitan areas to study ridehail adoption and use. Dillahunt et al. (2017) interviewed 12 low-income Detroit residents to study how they interact with ridehailing technology. Lee et al. (2015) interviewed ridehail drivers to better understand how they use the technology. Leistner and Steiner (2017) studied a Gainesville initiative to subsidize 1,445 ridehailing trips over 9 months for low-income seniors. Brown (2018) used data obtained from Lyft to analyze 6.3 million trips made by 828,616 people in Los Angeles. Brown (2018) also recruited passengers in Los Angeles to audit ridehail service equity there. Ge et al. (2016) deployed passengers in Seattle and Boston to make 1,500 trips to audit ridehail service equity in those cities. Wang and Mu (2018) in Atlanta, Hughes and MacKenzie (2016) in Seattle, and Thebault-Spieker et al. (2017) in Chicago accessed Uber API data to examine variations in the spatial distribution of wait times. Deka and Fei (2019) used the 2017 National Household Travel Survey (NHTS) to draw broad conclusions about the sociodemographics of ridehail adoption. Schaller (2018) also uses NHTS data combined with public utility and other data to study the typical ridehail user in 9 metropolitan areas. Finally, Alemi et al. (2018) used the 2015 California Millennials Dataset to study ridehail adoption and use.
The most statistically robust ridehail research has relied on analyzing large, aggregated, and anonymized data sets typically provided by the TNC under study. The broadest, most generalizable, ridehail research is based on recently released NHTS findings (Deka and Fei, 2019; Schaller, 2018). Though the NHTS lumped ridehailing use in with taxi and limousine use in its survey questions (FHA, 2016), rendering the disaggregation of implications for ridehail use dubious. Many other researchers have sought to survey and interview people about if and why they use ridehailing, compiling sample sizes from a few hundred to several thousand respondents.
Still, no research has presented findings from casually observing a small sample of individual ridehail passengers while comparing them to published literature. Yet similar observational methods have been applied in similar contexts. For example, Brown (2018) obtained ridehail driver age, gender, and race information as well as their working preferences through casual observation and volunteered information from a passenger’s perspective.
Common Ridehail Trip Types
Alcohol consumption is frequently cited as a strong motivating factor for ridehail use. Rayle et al. (2015) find 21% of ridehail users want to avoid drinking and driving. Henao (2017) finds that among those who drive, alcohol consumption and parking difficulties are top motivating factors; and among non-drivers, not having a car, poor transit, and time savings are top motivating factors for ridehail use. About a third of online survey respondents report to use ridehailing when avoiding drinking and driving, about the same share of those avoiding parking-related issues (Clewlow and Mishra, 2017). Gehrke et al. (2018) report the top 3 reasons for ridehail use are: because it is faster than transit, there is no car available, and to avoid parking hassles—in that order.
Work commuting is also a popular application of ridehail use. Henao (2017) reports that “most” non-drivers use ridehailing to get to work and school; and “most” drivers use ridehailing for social trips, going to the airport, or traveling while out of town. In their online survey Clewlow and Mishra (2017) found that “bars and parties’’ and “restaurants and cafes” made up 62% of trips. Across 3 studies, between 16% to 30% of respondents have reported using ridehailing for work commuting in the last 3 months (Henao 2017; Rayle et al. 2015; SUMC 2016). In Boston, Gehrke (2018) reports work trips top all other types, and Schaller (2018) finds 18% of trips in the 9 metros studied were for work commuting.
Ridehail Access and Equity
As ridehail use proliferates across both geography and socioeconomic status, it fills a transport gap for residents of some communities. In Austin, low-income users are more likely to use ridehailing for “utilitarian” purposes like work or school, and higher-income users are more likely to make social or airport trips (Dias et al., 2018). Low-income Los Angelenos made 25% more ridehail trips than their wealthier regional peers (Brown, 2018). Schaller (2018) found that very low-income households earning under $15,000 made more ridehail trips than middle-income earners. Bostonian ridehail users earning about the regional median income or less account for most weekly ridehail users (Gehrke et al., 2018). And Deka and Fei (2019) conclude that non-Hispanic Whites have a greater adoption rate yet are not likely to use ridehailing very frequently. Clewlow and Mishra (2017) found that White survey respondents had the smallest share of adoption with 21% having tried ridehailing. Black respondents had the greatest share of ridehail adoption with 27% who have tried it, followed by Asians at 23%, and Hispanics at 22%.
Though low-income and minority individuals may use ridehailing more frequently, they may also face barriers to access. Ge et al. (2016) report ridehail users with Black-sounding names have a relatively more difficult time getting a car to show up compared to users with White-sounding names. Brown (2018) also audited ridehail service equity and concluded similar results: Black users faced slightly higher cancellation rates than White users. However, Hughes and MacKenzie (2016) find essentially no relationship between race/ ethnicity and wait times in Seattle. Wang and Mu (2018) also find no association between race, income, and unemployment rate and wait times in Atlanta. Yet, Thebault-Spieker et al. (2017) conclude low-income residents in low density areas are forced to wait longer and pay more for a ridehail trip. Still, drivers have been found to turn off the ridehailing app while driving nearby “bad neighborhoods’’ to avoid receiving trip requests and “bad situations” (Lee et al., 2015). Surge prices are meant to incent more drivers to go online to meet rider demand, yet higher prices may edge out those low-income riders who depend most on ridehailing (Harding et al. 2016).
Getting Ready to Drive
Prior to the observations documented here, I had been driving for two months across both the Lyft and Uber applications. Saturday morning was selected for its relatively low frequency of trips (Brown, 2018), which provided a lower propensity for surge pricing that may have prohibited some would-be passengers. Based on my experience and research cited above, there is also no obvious trip type dominating Saturday morning ridehail travel, which should render passengers and their trip purposes less uniform. For example, sociodemographic and trip purpose uniformity is likely common among ridehailing trips at 1:00 a.m. on a Saturday morning—younger consumers of alcohol as cited above. Observations for this study were made on a sunny yet mild morning in March of 2019, which likely reduced the influence of weather on mode choice. Indeed, on that morning, there were no clear causes for exogenous shocks in ridehail travel demand or behavior.
Similar to how Servon (2017) researched the alternative banking industry by working as a payday-loan teller, I draw on first-hand casual observations and conversations with passengers from an Uber driver perspective. No other method allows for such a detailed study of how people and communities use and interact with ridehailing.
UberX ride requests were accepted beginning at 6:45 a.m. in Cincinnati’s central business district (CBD). Each subsequent trip request, based on the destination of the previous trip, was accepted without interference. For each incoming trip request the image of a generic human bust, as seen in Figure 6.1, was prominently displayed on the screen along with a few prospective trip details to aid the driver in deciding whether to accept or decline: driving time to pickup location, distance to pickup in miles, and average star rating of the requesting passenger. Other information sometimes included with applicable trip requests included: surge rates, pickup premium, and scheduled ride information. Trip details were overlaid onto a map containing the user’s origin and driver’s current location connected by a blue line representing the recommended travel route. The Uber application permitted the driver approximately 5 seconds to view the information and decide whether to accept the ride before the request expired and then presumably moved on to the next closest available driver. Time permitted for driver acceptance was graphically presented as a thin, white, diminishing circle encompassing the would-be passenger’s bust (Figure 6.1).
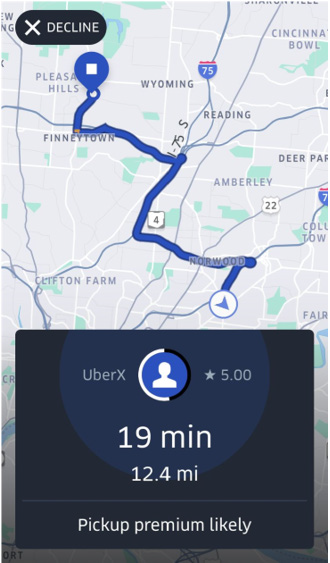
The information presented regarding gender, race/ethnicity, and age is based on my observation alone—they may not be correct. Trip purpose, reason for using Uber, and other details are based on observation and information volunteered by the passenger, rather than asking predetermined questions as one would in a survey or interview. Trip time, distance, and earnings were collected from the Uber driver application after the final ride was completed. Where appropriate and useful, I draw on previous experiences of completing more than 200 ridehail trips in Cincinnati as the driver.
Uber Everywhere
The intent here is to make an interesting addition to the ridehail literature by providing a first-hand driver’s account of typical passengers encountered on a typical day. So much of the published research on passengers slices and dices them into social and demographic silos while assuming each is the embodiment of homo economicus—masters of utility maximization. But in reality humans are messy, we make mistakes, we don’t know if it’s more polite to sit up front with the Uber driver or in the back alone. We sometimes miss the bus and have no choice but to Uber to work—and even though the cost of the ride may exceed an hour’s wages, the cost of not showing up is far greater. We are sometimes poor judges of when to talk, and when to be silent. And we are often bad tippers. The following attempts to capture some of that which is not easily sussed out in a survey, or measured in a data set. It is a timeline and narrative of the trips completed on that brisk March morning in Cincinnati, including those messy human details.
6:53 a.m.
After eight minutes of waiting for a trip request, the first one is a Black Millennial male waiting on a sidewalk in the CBD. He gets into the passenger side rear seat as most people do. He is in good spirits and explaining how rewarding it feels for him to be awake so early and on the move. It is not obvious whether he is a local—and where he was picked up from offered no clues. Neither does the purpose of his trip: across the CBD to a hotel restaurant to meet a friend for breakfast. He politely says thanks and de-cars. Time: 3 minutes 24 seconds. Distance: 0.62 miles. Driver’s take: $3.01.
7:07 a.m.
It took another ten minutes or so of waiting before the next trip request came in. It came from a neighborhood colloquially known as uptown—which is up a hill and dominated by students of the University of Cincinnati. A White Millennial male emerges from a single family home and enters the passenger side front seat. This does not happen often, so when it does, it is a bit surprising. A passenger from the previous day once told me that she sits in the front seat because to do otherwise would be rude. She also hinted that she really likes getting five star ratings and sitting up front may help in that effort. But this particular passenger does not seem concerned about his rating. He smells of alcohol and appears to be dressed in the previous day’s clothing, remarking that a first date the night before went pretty well. Dropped off at fraternity house near the university. Time: 2 minutes 30 seconds. Distance: 0.65 miles. Surge of $2.50, driver’s take: $5.51.
7:25 a.m.
There is often a lot of waiting around as a ridehail driver, well in Cincinnati on Saturday mornings there is anyway. Another fifteen minutes go by before another request comes in, this time from a rider in a neighborhood called Walnut Hills. A Black Millennial male emerges from an apartment building and enters the passenger side rear seat. He inquires about available employment because he dislikes his current job at an online shopping fulfillment center. Though right now he is making this trip to pick up a young girl of maybe 3 years old. After a short drive the first stop is another home in the same neighborhood. The passenger is in the home for about ten minutes while retrieving the child—any relation between the man, child, and this location would be speculation. Eventually, they both get in the car through the rear passenger door. He does not have a carseat for the child and neither does the driver, for the remainder of the trip the passengers do not use their seatbelts. On the drive back to where the ride began the young girl is scolded harshly for wetting herself. Both exit the car through the door they entered without comment. Time: 14 minutes 48 seconds. Distance: 2.34 miles. Driver’s take: $4.64.
7:51 a.m.
The fourth ride request comes after another ten minutes of waiting, this time it is from a nearby neighborhood named Avondale. A Black female Generation-X’er emerges from a single family home and enters the passenger side rear seat. She is upbeat, in a good mood, and on her way to work at a local nonprofit organization. She reveals through casual conversation that she usually drives her own car to work, but that is no longer possible because of a spell of bad luck. She states that on a recent weekend she had left her car in her employer’s parking lot, upon returning on Monday she found her car to be stripped of parts and left on blocks. She sold what remained to a junkyard and is currently carless. Stories of this sort are somewhat regular, sometimes people slip in and out of car-lessness even when they do everything right. She gets dropped off at the employee entrance—just off of the mostly empty parking lot. Time: 4 minutes 53 seconds. Distance: 1.62 miles. Surge of $3.50, driver’s take: $6.51.
8:05 a.m.
After another ten minute wait comes the fifth ride request, which was back in Uptown—the neighborhood generally surrounding the University of Cincinnati. A male international Millennial graduate student waiting on the sidewalk outside of a single family home enters the passenger side rear seat. Pleasantries are exchanged but the conversation remains somewhat superficial due to a language barrier. He states that usually he would walk, but today he awoke late and is in a hurry to get to campus. He gets dropped off at the university, and before exiting he shakes the driver’s hand. Time: 3 minutes 25 seconds. Distance: 1.03 miles. Driver’s take: $3.01.
8:17 a.m.
Trip six is also in the general Uptown vicinity, which is nice since no deadheading is required. A female international Millennial college student emerges from an apartment building carved out of a steep hill, she enters the passenger side rear seat. Again, a language barrier prohibited a meaningful discussion, though it was clear she was heading to campus to study. The driver’s homebrewed coffee aroma wafting from an insulated mug must have filled the car. She comments how much she enjoys the coffee smell and asks questions about the brewing process. She is dropped off at the university. Time: 5 minutes 2 seconds. Distance: 1.44 miles. Driver’s take: $3.19.
8:29 a.m.
Trip seven’s passenger was a White Millennial male waiting on the sidewalk among single family homes in that same neighborhood near the University of Cincinnati. He enters the car through the passenger side rear seat. He is heading home to the Hyde Park neighborhood on the city’s east side. He is quite friendly and talkative so conversation topics change quickly. Finally, one is settled on—he asks for an opinion on how to stop mass shootings. Takes are offered on what is believed to be at the core of the problem. Disagreement ensues. He is dropped off at an apartment building. Time: 11 minutes 30 seconds. Distance: 4.86 miles. Driver’s take: $8.36
8:59 a.m.
Trip seven ended in the moderately affluent and popular Hyde Park neighborhood, and trip eight’s passenger was based there too. A White Millennial male vaping on the sidewalk outside of a long row of beige two story apartment buildings enters my car through the passenger side front seat. He’s friendly and easy to talk to, which might help to explain two things: 1) why he sat in the front, and 2) his occupation as a car salesman. As a car salesman he previously sold the type of car in which he was riding—a Subaru Legacy. He offers to reveal a secret that all Subaru salesmen know, that the Legacy is the classiest of all Subarus. The cars he sells now are not as easy to sell. He was dropped off at a car dealership for work. He never vaped in my car, a gesture for which I was thankful. Time: 10 minutes 0 seconds. Distance: 3.45 miles. Surge of $6.25, driver’s take: $10.76.
9:10 a.m.
Trip nine came quickly after dropping off trip eight. A Black Generation-X female emerges from a single family home in a neighborhood near the car dealership, we are out of Hyde Park now. She enters my car through the passenger side rear seat. Conversation is light but friendly. She is on her way to work as a store manager at an indoor shopping mall— among the largest in the region. We talk about how she has a car and occasionally drives herself to work, but she has a “phobia” of driving so Uber is her preferred mode. She states that she tries to only treat herself to Uber when she “deserves it,” like after a long work week. She was dropped off at the mall entrance. Time: 6 minutes 30 seconds. Distance: 2.98 miles. Driver’s take: $3.65.
9:52 a.m.
The next request took over 30 minutes to come in. This one was from the Oakley neighborhood—a younger, edgier version of Hyde Park. A White Millennial female walking down a sidewalk in an apartment complex enters the driver side rear seat—an atypical seating arrangement. She is dressed in St. Patrick’s Day garb and heading to a friend’s apartment before heading out to partake in holiday festivities. She expresses concern nobody else will be similarly dressed and that she will be embarrassed. Ultimately, however, she is not too worried due to a pending move out of town to take over her father’s business. She was dropped off at an apartment building. Time: 10 minutes 51 seconds. Distance: 2.87 miles. Surge of $5.25, driver’s take: $9.65.
10:07 a.m.
Trip eleven is also on the east side of town, this time near the banks of the Ohio River from a newly built rowhouse designed to withstand rising waters. A White Millennial male emerges from a single family home and enters the passenger side rear seat. He is meeting family at a bar before engaging in St. Patrick Day festivities in Cincinnati’s CBD. He is friendly and jovial, in fact he even told a few “dad jokes.” He was dropped off at a downtown bar. Time: 10 minutes 52 seconds. Distance: 4.21 miles. Driver’s take: $5.11.
10:24 a.m.
Trip eleven ended downtown, which is where trip twelve was located. A German Generation-X male waiting on the sidewalk outside of a hotel enters the passenger side rear seat. He is talkative and curious about the city he’s visiting for business purposes. He is especially curious about the nuances of driving on downtown streets. The motivation behind his curiosity is eventually revealed by his trip purpose— to pick up a rental car. He also reveals that he is quite nervous to drive in the States. He remarks that the driver is not like most “cabbies” he’s known. He was dropped off at a car rental office, and left a $2 tip in the app. Time: 7 minutes 18 seconds. Distance: 1.05 miles. In-app tip of $2, driver’s take (including tip): $5.01.
Filling a Transport gap
For some passengers, ridehailing fills a critical gap in transport options, if only temporarily. Clewlow and Mishra (2017) find that 48% of ridehail and transit users do not personally own or lease a car. The example presented above of the woman whose car was stripped of parts is a particularly dramatic case of car-loss. More commonly, car-loss due to failed mechanics or the inability to afford the full cost of ownership (Chapple 2006) drive the carless to use ridehailing. Klein and Smart (2015) show that some residents of disadvantaged neighborhoods slip in and out of car ownership, affecting their ability to attain and maintain employment.
For other passengers ridehailing is a reprieve from driving. At the end of a long week of working overnight shifts, some ridehail passengers prefer not to drive themselves home—a sort of treat or self-reward. A distaste or even fear of driving, as presented above, is a common motivating factor for passengers to take ridehailing, even though a reliable car is available at home. Indeed, published survey results reflect these motivations (Henao, 2017; Clewlow and Mishra, 2017).
The case of a man picking up a child presented above provides an example of ridehail use that has extended beyond work commuting, to include other essential life tasks. Further, this case also presents a risk commonly borne by ridehail drivers: the decision of whether to terminate a trip (and loss of the fare) with either an unaccompanied minor, or accompanied minor without an appropriate car seat or restraints.
The Influence of Alcohol
Three, and likely more, of the Uber passengers presented above reflect findings that suggest alcohol consumption is a common motivating factor for ridehail use, even during hours not traditionally associated with alcohol consumption. The prevalence of alcohol in influencing ridehail use also conforms to nearly all published research, as cited in the literature review. However, the relationship between alcohol consumption and ridehail use is not monolithic. Actually, in this driver’s experience, it can manifest in three distinct trip scenarios made at different hours of the day. 1) This is the scenario commonly associated with alcohol consumption and ridehailing: the passenger who plans ahead to consume alcohol and makes the round trip by ridehailing. 2) The passenger who drove themselves (or arrived by other means) to the location of consumption, who then makes a return ridehail trip home at the conclusion of over-consumption. 3) This scenario is frequently related to the second: the passenger who makes a return ridehail trip to pick up a vehicle, or to place of residence, previously left at the location of over-consumption.
Driver’s Perspective
Demand for rides and the resulting revenue was unpredictable and seemingly random. Only one tip worth $2 on the twelfth and final ride was received, though tips are certainly more common in other service sectors. Additionally, four surge fares were scattered throughout the morning. Surges and the tip combined to make up nearly 30% of the $68.32 grossed over the four hours of driving. Without them less than $50 would have been earned, or a little more than $12 per hour, before deducting taxes and expenses. Many researchers have attempted to calculate the average hourly driver pay after accounting for all applicable costs, including: vehicle maintenance, depreciation, fuel, and insurance. Wildly different findings have been published, and earnings vary by region. Still, two papers studying Seattle driver earnings published just days apart report drivers average $23.52 per hour (Hyman et al. 2020) and $9.73 per hour (Parrott and Reich 2020)—both calculated to be after expenses are paid.
At the time this research was conducted, ridehail drivers in Ohio did not receive additional training and were not required to obtain special license endorsements. Because of this, drivers may unwittingly assume undue risk by not being fully knowledgeable of applicable laws, sometimes between multiple states. The Cincinnati ridehail market covers most of Southwest Ohio, regions of Northern Kentucky, and regions of Southeastern Indiana. It seems reasonable to assume that a significant proportion of ridehail drivers are unfamiliar with the current state of regulatory affairs in each jurisdiction. Indeed, there often seems to be a disconnect between social norms and regulation. For example, many passengers presented failed to fasten their seatbelts for the duration of the trip, whether seated up front or in the back.
Discussion
This work aims to supplement quantitative ridehail research by presenting qualitative findings obtained through observation and casual conversation. The intent is to place examples of ridehail passengers and the transport decisions they make within the context of current academic knowledge. A fine point is added to each ride account by presenting small and sometimes quirky details about the trip. This is done to provide additional insights into the character of ridehail passengers, their reasons for using ridehailing, and the unique social relationship between ridehail drivers and passengers.
Interestingly, the two passengers who sat up front with the driver were White Millennial males. This could be because I, too, am a White Millennial male. For female passengers, sitting within easy reach of the driver may be a safety concern leading them to sit directly behind the driver. It could also be a reflection of the varying role ridehailing plays in the lives of passengers.
This work has implications for researchers and policymakers alike by presenting details about trips and passengers not seen before in the literature. For example, international college students have few options for commuting to school and may depend on ridehailing. For some, ridehailing may provide a preferred work commute experience over transit and driving while adding a layer of transport reliability. For social trips, alcohol consumption manifests in several different types of ridehail trips. For drivers, dependence on the extra revenue from tips and surges is unreliable and unpredictable yet necessary. Also, as the driver is presented trip origin, suggested route, star rating, and sometimes a photo of the passenger before accepting a ride, would-be riders remain vulnerable to biases and discrimination.
References
Alemi, F., Circella, G., Handy, S., & Mokhtarian, P. (2018). What influences travelers to use Uber? Exploring the factors affecting the adoption of on-demand ride services in California. Travel Behaviour and Society 13: 88-104.
Brown, A. E. (2018). Ridehail Revolution: Ridehail Travel and Equity in Los Angeles. PhD diss., Luskin School for Public Affairs, University of California at Los Angeles. Available at: https://escholarship.org/uc/item/4r22m57k.
Campbell, H. (2015). My Rating System for Uber and Lyft Passengers. The Rideshare Guy. Available at: https://therideshareguy.com/my-rating-system-for-uber-and-lyft-passengers/. Accessed May, 2018.
Chapple, K. (2006). Overcoming mismatch: Beyond dispersal, mobility, and development strategies. Journal of the American Planning Association 72 (3): 322-36.
Clewlow, R. R., & Mishra, G. S. (2017). Disruptive Transportation: The Adoption, Utilization, and Impacts of Ride-Hailing in the United States. Davis, CA: Institute of Transportation Studies, University of California, Davis. Available at: https://itspubs.ucdavis.edu/index.php/research/publications/ publication-detail/?pub_id=2752.
Deka, D., & Fei, D. (2019). A Comparison of the Personal and Neighborhood Characteristics Associated with Ridesourcing, Transit use, and Driving with NHTS Data. Journal of Transport Geography 76: 24-33.
Dias, F. F., Lavieri, P. S., Kim, T., Bhat, C. R., & Pendyala, R. M. (2018). Fusing Multiple Sources of Data to Understand Ride-Hailing Use. University of Texas, Austin, TX. Available at: http://www.caee.utexas.edu/prof/bhat/ ABSTRACTS/RidehailingUsersDataFusion.pdf.
Dillahunt, T. R., Kameswaran, V., Li, L. &, Rosenblat, T. (2017). Uncovering the Values and Constraints of Real-time Ridesharing for Low-resource Populations. CHI 2017 conference paper, May 6-11, 2017, Denver, CO, USA.
Ge, Y, Knittel, C. R., MacKenzie, D., & Zoepf, S. (2016). Racial and Gender Discrimination in Transportation Network Companies. NBER Working Paper 22776. Available at: http://www.nber.org/papers/w22776.pdf.
Gehrke, S. R., Felix A., & Reardon. T. (2018). Fare Choices: A Survey of Ride- Hailing Passengers in Metro Boston. Metropolitan Area Planning Council. Available at: https://www.mapc.org/farechoices/.
Harding, S., Kandlikar, M., & Gulati, S. (2016). Taxi apps, regulation, and the market for taxi journeys. Transportation Research Part A 88 : 15-25.
Henao, A, (2017). Impacts of Ridesourcing – Lyft & Uber – on Transportation Including VMT, Mode Replacement, Parking, & Travel Behavior. PhD diss., Civil Engineering Program, University of Colorado at Denver. Available at: http://digital.auraria.edu/content/AA/00/00/60/55/00001/Henao_ ucdenver_0765D_10823.pdf.
Hyman, L., Groshen, E. L., Litwin, A. S., Wells, M.T., & Thompson, K.P. (2020). Platform driving in Seattle. Ithaca, NY: Cornell University, ILR School, Institute for Workplace Studies.
Hughes, R., & MacKenzie, D. (2016). Transportation network company wait times in greater Seattle, and relationship to socioeconomic indicators. Journal of Transport Geography 56 : 36-44.
Klein, N. J., & Smart, M. J. (2015). Car today, gone tomorrow: The ephemeral car in low-income, immigrant and minority families. Transportation 44 (3): 495- 510.
Lee, M. K. Kusbit, D., Metsky, E., & Dabbish, L. (2015). Working with machines: The impact of algorithmic and data-driven management on human workers. In Proceedings of the 33rd Annual ACM Conference on Human Factors in Computing Systems. ACM, 1603–1612. DOI:https://doi. org/10.1145/2702123.2702548.
Leistner, D. L, & Steiner. R. L. (2017). Uber for seniors? Exploring transportation options for the future. Transportation Research Record (2660): 22-9. NHTS. 2017. 2017 National Household Travel Survey. Edited by U.S. Department of Transportation FHA.
Parrott, J. A., & Reich, M. (2020). A Minimum Compensation Standard for Seattle TNC Drivers. Available at: https://irle.berkeley.edu/files/2020/07/ Parrott-Reich-Seattle-Report_July-2020.pdf.
Rayle, L., Dai, D., Chan, N., Cervero, R, & Shaheen, S. (2015). Just Better Taxi? A Survey-Based Comparison of Taxis, Transit, and Ridesourcing Services in San Francisco. Transport Policy 45: 168–78. Saturday Night Live. 2016. Uber for Jen. NBC Universal Studios. (https://www.youtube.com/ watch?v=aaVBoEeDK4Q)
— (2017). Five Stars. NBC Universal Studios. (https://www.youtube.com/ watch?v=sDQaSuikPss&t=87s)
Schaller, B. (2018). The New Automobility: Lyft, Uber and the Future of American Cities. Brooklyn, NY: Schaller Consulting. Available at: http://www. schallerconsult.com/rideservices/automobility.pdf.
Servon, L. J. (2017). The unbanking of America: How the new middle class survives. Boston, MA: Houghton Mifflin Harcourt. Interaction (TOCHI) 24 (3): 1-40.
Thebault-Spieker, J, Terveen, L., & Hecht, B. (2017). Toward a geographic understanding of the sharing economy: Systemic biases in UberX and TaskRabbit. ACM Transactions on Computer-Human Interaction (TOCHI) 24 (3): 1-40.
Wang, M. S, & Mu, L. (2018). Spatial disparities of Uber accessibility: An exploratory analysis in Atlanta, USA. Computers Environment and Urban Systems 67: 169-75.