
Abstract: Cities require finer-scale climate information for resilient infrastructure development and adaptation planning, beyond standard climate analysis. Urban downscaling, a complex and computationally intensive process, involves generating detailed climate data for cities and neighborhoods from coarser sources. This study presents the ‘DownScaleBench,’ a novel deep learning approach facilitating urban downscaling to any location. Utilizing ground-based and satellite/reanalysis data, it employs an iterative super-resolution convolutional neural network (Iterative SRCNN) to enhance resolution. Applying this method produces a high-resolution (300 m) precipitation dataset from a coarse (10 km) satellite-based product (JAXA GsMAP). Validation against in-situ observations during heavy rain events in Austin, Texas, demonstrates significant improvement over baseline cubic interpolation. The DownScaleBench shows promise for creating high-resolution urban meteorological datasets, essential for climate-resilient city planning.
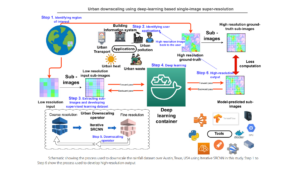
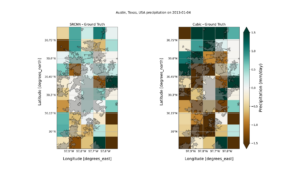
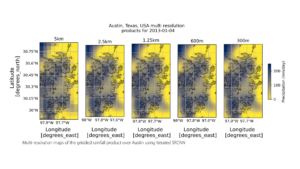
For more information, please visit: https://link.springer.com/article/10.1007/s43762-023-00096-9