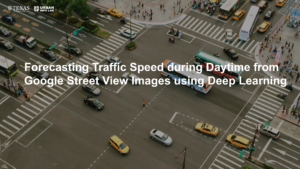
Abstract: Traffic forecasting is vital for urban planning, with deep learning methods excelling in capturing traffic patterns. However, obtaining comprehensive historical data remains challenging, especially for city-wide predictions. To overcome this, we used SceneGCN, a deep learning approach, for city-scale traffic speed forecasting. This method involves extracting scene features from Google Street View (GSV) images using pretrained Resnet18 models, followed by utilizing a graph convolutional neural network to predict traffic speed at different times of day. Our results show that the model achieves up to 86.5% accuracy, with Resnet18 pretrained by Places365 being the best choice for feature extraction. This study underscores the effectiveness of predicting city-scale traffic speed using GSV images, indicating their potential in capturing human activity details.
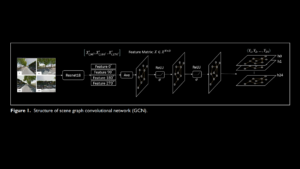
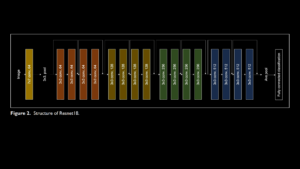
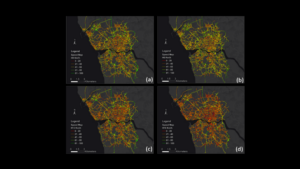
For more information, please visit: https://journals.sagepub.com/doi/10.1177/03611981231169531?icid=int.sj-full-text.similar-articles.7