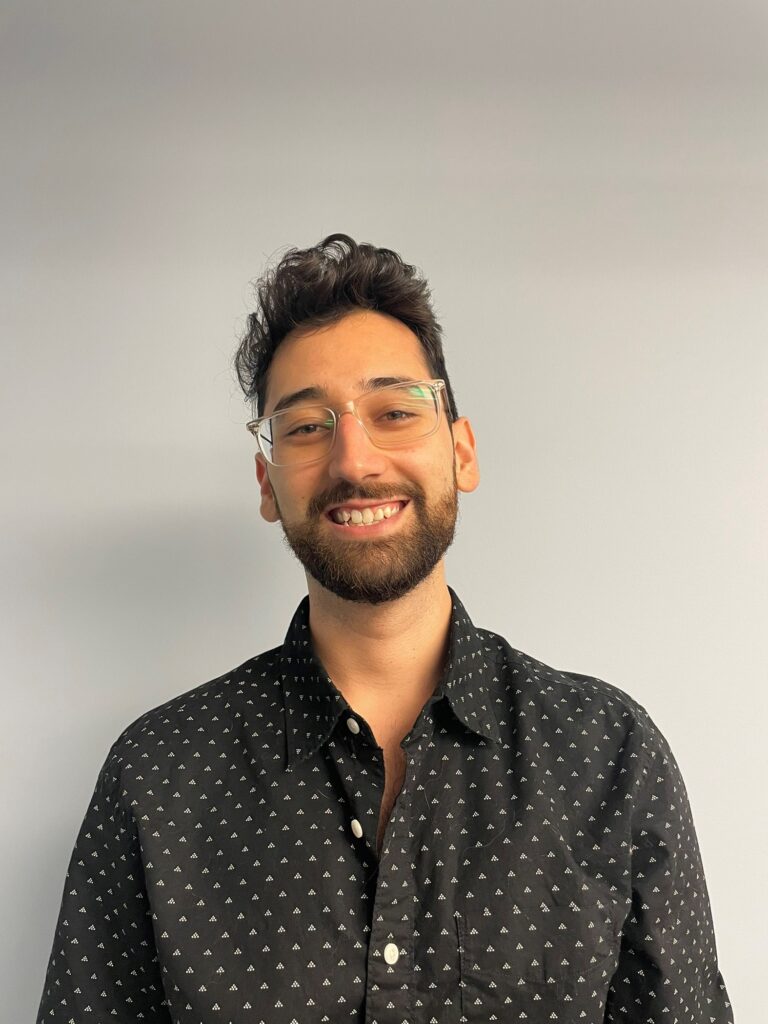
Jorge Cisneros Paz (he/him/his) is currently a postdoctoral researcher in the Department of Biomedical Engineering at the University of Texas at Austin. He received his PhD degree in June 2022 from the Department of Applied Mathematics at the University of Washington, where he also earned his MS degree in 2019. His dissertation addresses the complications that arise in finite-difference methods with boundary conditions and ghost points when solving nonlinear problems. In 2017, Jorge received BS degrees in mathematics and physics with a minor in chemistry from the University of Texas Rio Grande Valley. Jorge’s research efforts focus on developing numerical techniques to solve problems that arise in all fields of STEM, ranging from dispersive phenomena to optimization methods to reduced-order methods. He has participated in many research programs since his undergraduate years, including a recent 10-month internship with the biotech company Genentech, and has presented at over 30 conferences with travel awards. Jorge was recently awarded an NCI Diversity Supplement Grant and the MGB-SIAM Early Career Fellowship. In addition, Jorge is an ORISE Fellow working on projects related to privacy data methods with the National Science Foundation and the US Census Bureau.
At DMIC, Jorge is developing deep-learning methods for biomedical image modalities and their applications, collaborating with several other labs across the department. Primarily, he is exploring how deep generative models can be leveraged with optimization methods for lung 3DCT artifact correction and dose distributions for functional avoidance radiotherapy. Jorge also runs the AI Medical Imaging Seminar geared towards our department students and postdocs interested in applications at the intersection of AI and biomedical imaging.