”Failure is success if we learn from it.” -Malcolm Forbes
RESEARCH AREA
- Extreme Coastal Hazards – Investigating the impacts of storm surges, tsunamis, sea-level rise, flash floods, high-tide flooding, and compound flooding.
- Coastal Resilience & Adaptation – Enhancing resilience and public health to climate-driven coastal risks through multi-hazard modeling and sustainable adaptation strategies.
- Vulnerability & Risk Assessment – Assessing infrastructure damage, evacuation dynamics, and coastal risk hotspots using digital twins and data-driven simulations.
- Coastal & Nearshore Processes – Studying barrier island dynamics, sediment transport, and nearshore hydrodynamics to improve coastal hazard mitigation.
- Nature-Based Solutions for Coastal Protection – Developing and evaluating ecosystem-based strategies to reduce risks and enhance resilience in coastal and ocean environments.
RESEARCH TOOLS
We tackle exciting science and engineering challenges associated with extreme hazards in coastal and ocean environments. By combining numerical modeling, machine learning, and remote sensing, we develop innovative solutions to address these issues. Here are some of the tools we use:
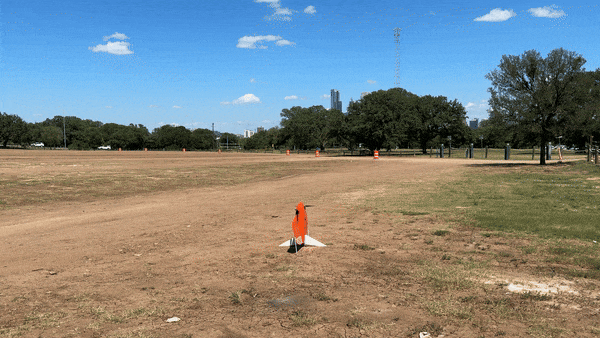
Remote Sensing Tools (Drones and Satellites)